MIMOSAS
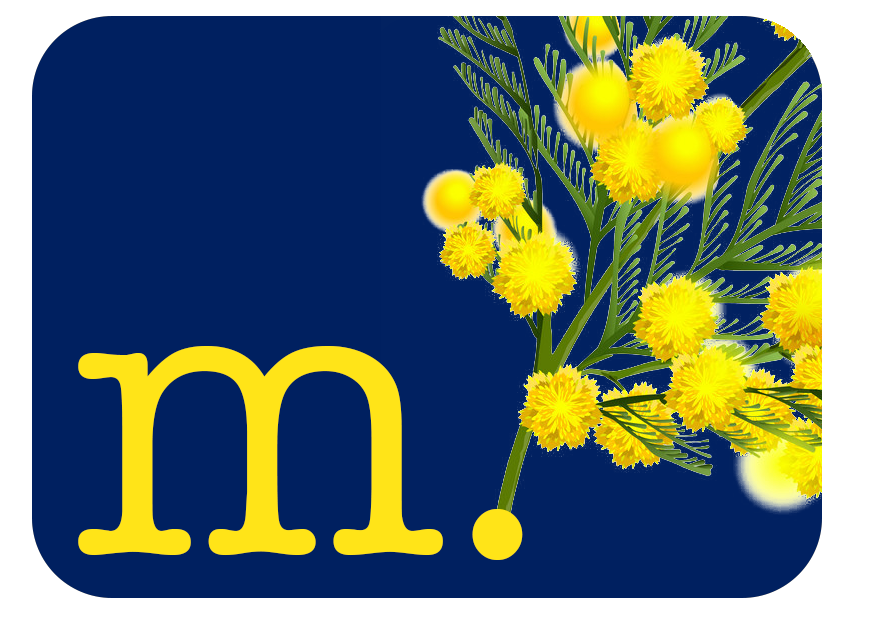
Multisource Input Model Output Security Analysis Suite
Github
Get the CodeManual
Read the ManualDocumentation
Get the DocsAbstract
A supervised machine learning pipeline, MIMOSAS (Multisource Input Model Output Security Analysis Suite), has been developed for classification of multimodal data to inform nuclear security and proliferation detection scenarios. MIMOSAS provides an end-to-end data processing workflow, from data ingestion and pre-processing to model training and test set classification. The pipeline is specified via an input deck, making workflow customization effortless, and the framework is modular allowing for the easy addition of new learning algorithms. In the current build, the user selects from decision tree, random forest, and feed-forward neural network classifiers to train customizable models with built-in cross validation methods for hyperparameter optimization. Trained model outputs are stored with the associated metadata for rapid deployment. These can be applied in supervised classification to assess previously unseen data or for further training as new observations are added to the existing data set. MIMOSAS provides the capability to fuse a wide range of data sources (e.g., radiation, environmental, acoustic, seismic, imagery, etc.) to make, confirm, and correlate machine learning predictions for nuclear security applications.
Cite This Work
Publications that use MIMOSAS should cite the software as follows:
Jared Zhao, Bethany L. Goldblum, Christopher Stewart, Alicia Ying-Ti Tsai, Shruthi Chockkalingam, and Pedro Vicente Valdez. (2019). MIMOSAS: Multimodal Input Model Output Security Analysis Suite (Version 1.0) [Computer software]. Berkeley, CA: University of California, Berkeley.
Contact
Bethany L. Goldblum
UC Berkeley